AI Adoption Case Study: AVEVA's work with Drax and SUEZ on AI driven predictive maintenance
techUK’s AI adoption collection of case studies showcases examples of how organisations are Putting AI into Action, either through the adoption of AI models within their organisations, or by developing AI tools that can be leveraged by others.
By shining a light on these use cases, techUK hopes to demonstrate examples of best practice from across sectors and from organisations of all sizes.
1. Challenge:
Drax are one of the top producers of low carbon electricity in the UK, while SUEZ manages and processes household waste for a large number of local authorities, endowing it with a key role in the circular economy. Both companies have a major part to play in the country’s transition to Net Zero.
Latent degradation and issues with high integrity industrial equipment in Drax and SUEZ plants such as boilers, turbines, pumps and generators can grow into defects which eventually cause unplanned downtime, interrupting the reliable production of power and the processing of waste. Large industrial plants are fitted with tens of thousands of IoT sensors measuring temperatures, pressures, flows, vibrations the recording huge amounts of data minute by minute every day. Over the past two years Drax and SUEZ both worked with AVEVA to deploy a complete diagnostics process harnessing that data to reveal the hidden heartbeat of their equipment health.
2. Solution:
Time series data historians such as AVEVA’s Pi system constantly store the data coming from equipment sensors, organise and contextualise the data and performing checks to ensure data quality. Predictive Analytics, a key AI enabling technology harnesses that data using an easily explainable machine learning anomaly detection model to encode the good operational behaviour of a piece of equipment from the historical sensor data. Once that model is deployed on the live data it is silently watching, twenty four hours a day and seven days a week, for the first hints of when an equipment departing from the good historical envelope. This gives warning, long in advance, and allows Drax and SUEZ to plan, assemble spare parts and schedule maintenance interventions when they will be least impactful to electricity production or waste processing. The solution is configured for Engineers and equipment experts to generate valuable outcomes; not only does it detect anomalies in the data, but it recognises signatures of typical faults, for example a damaged bearing, giving prescriptive advice for Engineers to be able to follow up with the appropriate corrective actions. When an anomaly is caught the system is gives precise citations to show the user which sensors are contributing such that the results of the system are highly accountable and not obscured in an AI ‘black-box’. The system is highly scalable capable of monitoring hundreds or thousands of pieces of critical industrial equipment, but is computationally highly efficient requiring little more than a desktop computer to run.
3. Impact:
From the first implementation of the software both Drax and SUEZ started to detect issues with their equipment.
Drax found a generator transformer gas in oil measurement increasing indicative of a serious fault. A similar transformer had previously failed causing an unplanned stop of the unit for more than four weeks. With the early detection provided by Predictive Analytics Drax were able to operate the transformer differently, perform a short remedial repair and continue operation until the next major planned stop.
One of SUEZ steam turbines showed signs of a large axial movement of the rotor; a tell-tale sign of a balance-piston seal failure. When the machine was later opened, the inspection verified that the seal was indeed severely damaged. Although this case didn’t pose an immediate risk to operation, the early detection allowed better preparation for the next planned overhaul for the risk that more work would be required to remediate.

Usman Ikhlaq
Usman joined techUK in January 2024 as Programme Manager for Artificial Intelligence.
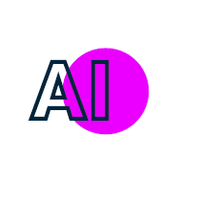
techUK - Putting AI into Action
techUK’s Putting AI into Action campaign serves as a one stop shop for showcasing the opportunities and benefits of AI adoption across sectors and markets.
During this campaign, techUK will run a regular drumbeat of activity, including events, reports, and insights, to demonstrate some of the most significant opportunities for AI adoption in 2025, as well as working with key stakeholders to identify and address current barriers to adoption.
Visit our AI Adoption Hub to learn more, or find our latest activity below.
Upcoming AI Adoption events
Latest news and insights
Sign-up to get the latest updates and opportunities across Technology and Innovation & AI.
Contact the team
Learn more about our AI Adoption campaign:
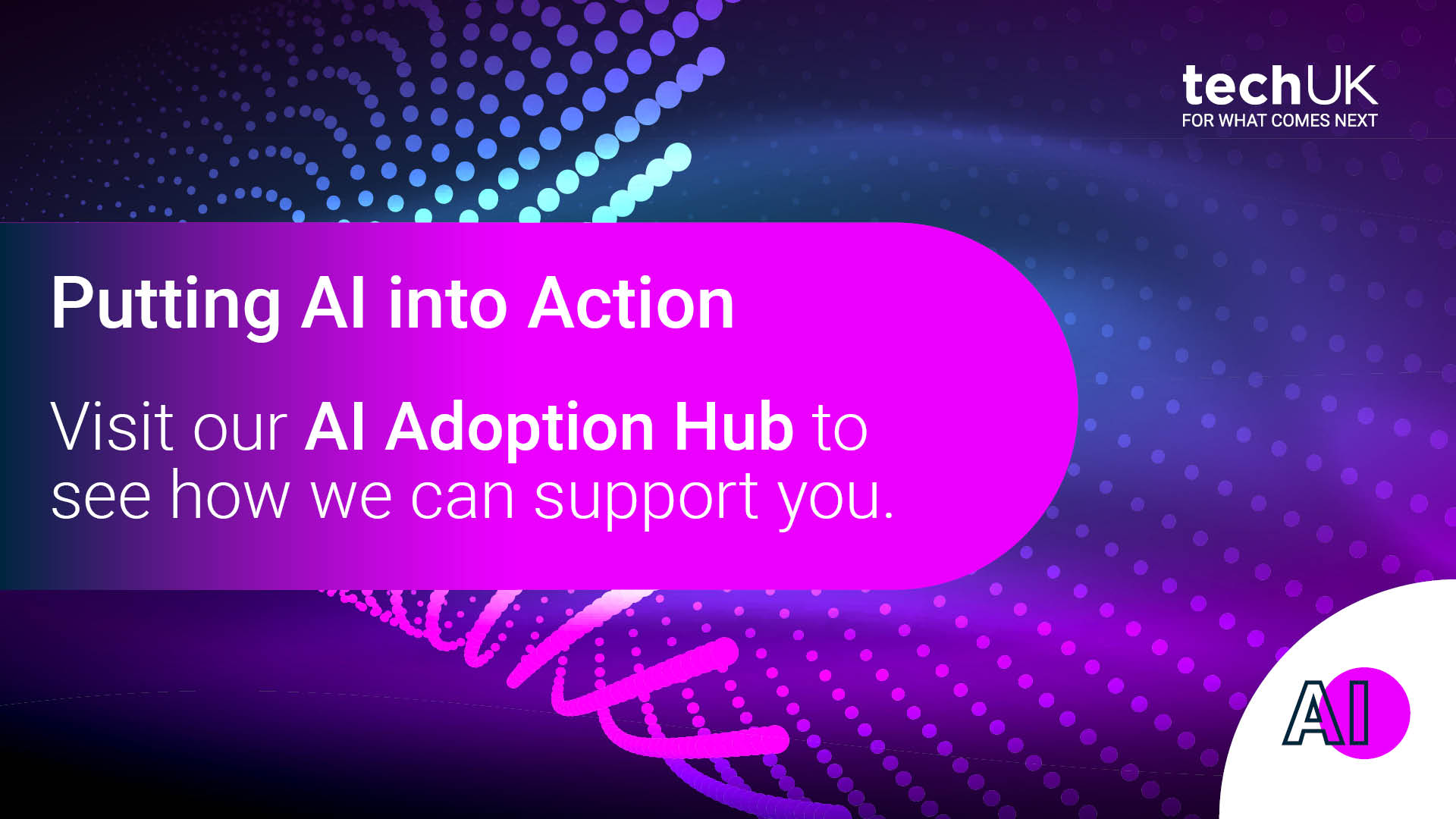