Data Analytics to improve detection of vegetation encroaching on power lines
Summary of Problem
Electricity distribution companies have a perpetual problem with vegetation growth and survey their networks frequently to ensure electricity lines and trees are kept apart. There are several reasons for doing this: trees whip in the wind and break cables, electricity can leak to ground through trees, arcing can set fires, and electricity regulators impose significant fines when supply is lost (of the order of £175m pa in the UK). In short, it’s in everyone’s interest to keep a tidy house but the very nature of the problem means it can only be controlled, never solved.
Historically, power distribution companies surveyed the network manually but this is cumbersome, expensive work which takes so long that results are often outdated and geographic records are often inaccurate leading to poor crew time-management in the field. To give an idea of the size of the problem, there are 800,000 km of power lines in the UK and globally there may be around 100 million km of lines.
Perhaps best known for its application in the autonomous vehicle sector, LiDAR is increasingly being deployed from planes, helicopters and drones as a quick and accurate way to survey power networks. LiDAR uses reflected LASER beams to build a 3D map of the surveyed area and geospatial awareness can be added by surveying the area from different angles. LiDAR provides a vast amount of data (called point-cloud data) - and a vast amount of data is what data analytics lives for.
LiDAR can easily be presented in graphical form suitable for humans to interpret but poring through the pictures to find anomalies is labour intensive, slow and boring work - inevitably leading to errors.
Data Analytics companies have been studying this problem for a while as it’s easy to see the advantages offered by AI and a much better solution is to leverage Data Analytics algorithms to detect anomalies in the data signals in the point clouds caused by encroaching vegetation. Companies who can join advanced AI vegetation detection algorithms with the scale of the public cloud and flexibility of technologies such as Kubernetes are able to scale up and down quickly and flexibly to match compute power with the size of the task required.
The results are available in a fraction of the time it can be done by humans, they’re more accurate and costs are dramatically lower. AI companies have used such techniques to reduce the time for what previously took several weeks or months to under 6 hours – quick enough for a crew to be scheduled to tackle problems the day following the survey with appropriate geo codes automatically derived from object classification.
Homomorphic techniques (whereby encrypted data is analysed without it first being decrypted) are used to ensure valuable or secret geospatial data is never exposed as the AI processor does not need to decrypt the data, and only the data owner is able to decrypt the results. An additional layer of security can be applied through an irrefutable chain of custody, proving who has had access to the data.
Future Developments
The imminent increase in the number of low earth orbit satellites equipped with LiDAR is expected to provide a rich source of high density point clouds suitable for analysis by AI. Applications extend well beyond vegetation detection, as the same algorithms that detect anomalies in the data signals from point-clouds can detect gas, battlefield weapons and even buried items such as landmines and IED’s so that large areas can be cleared and made safe. This is an exciting technology that promises to deliver benefits in so many areas.
About the author:
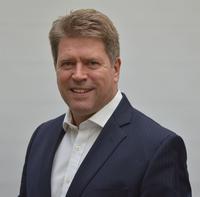
Nick Evans is the COO of Lockular Limited, a company specialising in securing data through a patented and trade-secret protected invention which not only protects the data but provides an irrefutable chain of custody with a record written to blockchain of anyone who has accessed, or has even attempted to access, the data.
Email: [email protected]
Twitter: @lockular

Katherine Holden
Katherine joined techUK in May 2018 and currently leads the Data Analytics, AI and Digital ID programme.