Measuring Impact: Causal analysis of Medical Science Liaisons satisfaction
The work of the medical science liaison (MSL) is becoming increasingly important in the pharma industry thanks to the critical role they play, independent of commercial activities. These personnel sit at the heart of pharma’s web of stakeholders and technical experts, acting as a conduit through which medical and scientific information can be exchanged with key opinion leaders (KOLs). This knowledge can significantly influence how a company manages its drugs portfolios and over the last decade a range of multinational and domestic pharmaceutical firms in Japan have invested heavily in teams of MSLs.
While surveys have frequently been used to quantitatively assess companies’ MSLs in terms of satisfaction and impact, there has been limited understanding of what the key drivers of these elements are. In this article, we will explore how combining domain expertise with causal analysis allowed QuantumBlack to explore the complex relationships between these outcomes and their drivers.
Survey results: A wide variance in MSL satisfaction
Over the past five years, McKinsey has conducted an industry-wide Medical Affairs Performance Evaluation Survey (MAPES) in Japan to assess how effective certain MSLs are. In January through February 2020, we conducted an online survey of over 200 KOLs in five disease areas (lung cancer, asthma/COPD, cardiovascular, rheumatology and dermatology) to understand how KOLs perceive pharma companies’ MSL interaction, clinical data and digital engagement.
From the 1,200 responses, the survey revealed that the perceived impact and level of satisfaction of MSLs varied widely across companies (Exhibit 1), which aligned with previous survey results. We also observed variance across companies in other metrics that we surveyed, such as satisfaction with objectivity of information provided and medical knowledge.
The survey results allow companies to see how their MSLs’ performances are perceived compared to others, but do not directly show what the key drivers of satisfaction and impact are. To discern these important elements, and how MSLs can improve, we needed to undertake causal analysis.
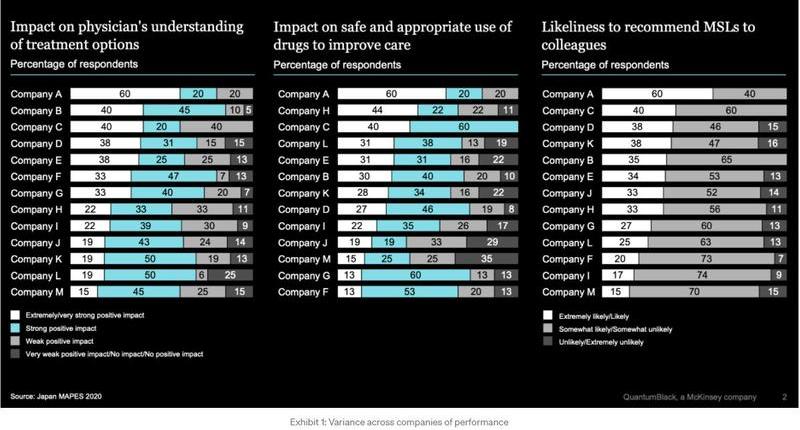
What can we find from causal analysis?
Causal analysis leverages Bayesian networks which, with the domain experts input, can work as a Structural Causal Model. This type of model is distinctive for two main reasons:
- The causal relationships can be easily visualised as a network structure. This incorporates domain expertise easily and efficiently.
- The structure of the network accounts for confounding factors. This hence unlocks complex ‘what-if’ counterfactual analysis which allows us to estimate the effect of interventions on the condition.
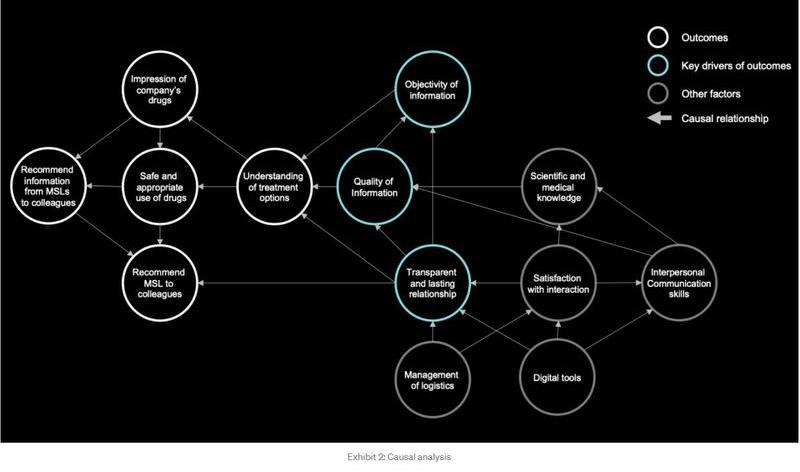
Causal analysis: Quality and objectivity of information and relationships drive MSL outcomes
We used Bayesian networks and machine learning techniques to model the relationship between outcomes and drivers. Compared to traditional correlation analysis among those factors, Bayesian networks, with the help of domain knowledge, can incorporate the causal relationship and their dependencies. The causal analysis was performed using CausalNex, an open source causal analysis toolkit developed by QuantumBlack Labs, leveraging the latest techniques from industry and academia.
Causal analysis of survey results (Exhibit 2 — a simplified visualisation without nodes that do not directly or indirectly drive outcomes) found that the key drivers (shown in turquoise) of the outcomes (shown in white) were:
- Objectivity and quality of information — both of these factors lead to physicians’ better understanding of current treatment options. This positively impacts a physician’s impression of the company’s drugs and enhances the physician’s safe and appropriate use of the drugs, which ultimately improves patient outcomes.
- Transparent and lasting relationships — this positively impacts physicians’ trust in the information provided by MSLs, enhances their understanding of treatment options and increases their willingness to recommend MSLs to their colleagues and peers.
These outcomes do not come as a surprise for many. When we asked survey respondents the unique value MSLs provide and what they could do to provide greater value, many replies focused on quality information and objectivity.
The importance of relationships is less immediately obvious. For example, in a recent large-scale reorganisation in a pharma company, the company discontinued or reallocated many relationships between physicians and the company. Within six months, there was an unprecedented drop in the overall impression of this company’s drugs and the willingness to recommend their MSLs. Even for an organisation with a decades-long presence in the specific disease area, underestimating the importance of relationships cost them significantly.
Use of appropriate analytics — why causation?
Interpretation of analyses using traditional techniques without domain experts can be limiting and at times can even be inadvertently misconstrued. For example, correlation analysis is often used to identify the top correlated factors with outcomes and then adapt these factors to improve outcomes. However, correlation does not imply causation, and without careful interpretation of domain experts can result in an incorrect conclusion.
In correlation analysis of our benchmark survey results, satisfaction with MSLs in providing information around company-sponsored opportunities (e.g. research) is the third most correlated factor with the impact on safe and appropriate use of drugs. However, it is logically not necessarily a driver for safe and appropriate use of drugs. In the more advanced causal analysis, the algorithm was able to visualise the relationships among variables, enabling domain experts to identify that information around company-sponsored opportunities is not an effective driver to improve safe and appropriate use of drugs in this situation.
Appropriate use of advanced analytics gives companies a holistic view and unlocks the true value of their data. Through using the CausalNex toolkit and working closely with domain experts, the analytics team were able to venture beyond correlation into the realm of causal relationships. Through identifying the causal drivers of the outcomes, the impact of each MSL became much clearer and highlighted areas for improvement.
This article was first published on QuantumBlack’s Medium account
Authors:
Matt McDevitt, COO, QuantumBlack Japan; Raymond Chan, Partner, Minyoung Kim, Partner, Tomoko Nagatani, Capabilities & Insights Specialist, Jan van Overbeeke, Partner, McKinsey & Company
The authors wish to thank Paul Beaumont, Shuhei Ishida and Xi Liang for their contributions to this article.

Katherine Holden
Katherine joined techUK in May 2018 and currently leads the Data Analytics, AI and Digital ID programme.