With great power comes great responsibility: the importance of proactive AI risk management
Organisations looking to implement AI technology are paying more attention to its risks. Last year, our Global Responsible AI Survey confirmed priority is currently given to AI risks identification and accountability within companies mainly via strategies and policies and an enterprise approach to AI. Four in ten companies (37%) have a strategy and policies in place to tackle AI risk which are communicated, a stark increase from 2019 ( 18%). One quarter of companies have an enterprise approach to AI risk which is communicated and standardised.
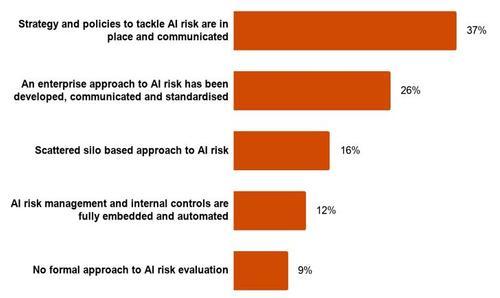
Figure 1: How companies identify AI risks (Source: PwC Responsible AI Survey)
While the increase in focus on AI risks is a positive sign, the adoption varies considerably among different companies sizes. Smaller companies, <$500m, where a lot of AI innovation happens, are more likely to have either no formal approach to AI (12%) risks or have a scattered approach to AI risk (21%), compared with large companies, <$1bn (6% and 14% respectfully) where there is a high degree of maturity, in terms of both resources, capabilities and knowledge, in managing risks overall.
In order to start managing the risks of AI in a consistent and sustainable manner, organisations of all size building and using AI, need to look beyond individual behaviours and adopt an ethics-by-design culture that would allow for proactive risk mitigation. We’ll illustrate this by exploring three of the biggest potential AI risk areas:
Performance risks Imagine your new AI solution for processing credit applicants appeared to be rejecting candidates of particular backgrounds - how confident would you be in explaining why that decision was made? In the last few years, the awareness of risks associated with bias in data, algorithms and the ‘black box’ effect has grown, fostering significant research aimed at reducing model bias and increasing model stability. This is particularly important when AI solutions are implemented at scale. Retaining a certain number of humans ‘in the loop’ to audit algorithm outputs can be an effective way to mitigate this.
Security risks Some learning AI models can be susceptible to malicious actors who provide false input data, intended to create spurious outputs (eg intentional mistraining of chatbots). One way to mitigate this is to simulate adversarial attacks on your own models and retrain them to recognise attacks; these are activities which should be considered in the design phase of the model.
Control and governance risks Even today, algorithms that could be classified as ‘narrow-AI’ (designed to perform one specific task), can sometimes yield unpredictable outcomes that lead to unanticipated and harmful consequences.Good governance (eg hard controls, regular model challenge, surrogate explanation models etc) is required to build explainability, transparency and controls into the systems.
To mitigate AI risks at an organisational level you need to account for the maturity of the existing risk management framework within the organisation, the industry it operates in, the levels of risk implicit in the use-cases and the relevant regulatory environment. With these controls in place, enterprises are in a position to consistently identify and manage risks at every stage of the AI lifecycle.
Two critical questions on AI risks that should be part of your strategic planning:
- What are the most important AI threats and opportunities to your organisation? Are there plans to identify and address them? Are the current business models , organisational structures and capabilities sufficient to support the organisation’s desired AI strategy?
- Are the roles and responsibilities related to strategy, governance, data architecture, data quality, ethical imperatives, and performance measurement clearly identified?
AI has huge potential to deliver beneficial business and societal outcomes. However, this requires AI systems to be designed and implemented with fairness, security, transparency and control in front of mind. The most efficient method to identify and manage AI risks is ultimately with proper AI governance.
Author:
Maria Axente, Responsible AI and AI for Good Lead, PwC United Kingdom
You can read all insights from techUK's AI Week here